Chasing Chaos: Can Bittensor's AI Oracles Decode Mandelbrot's Market Fractals?

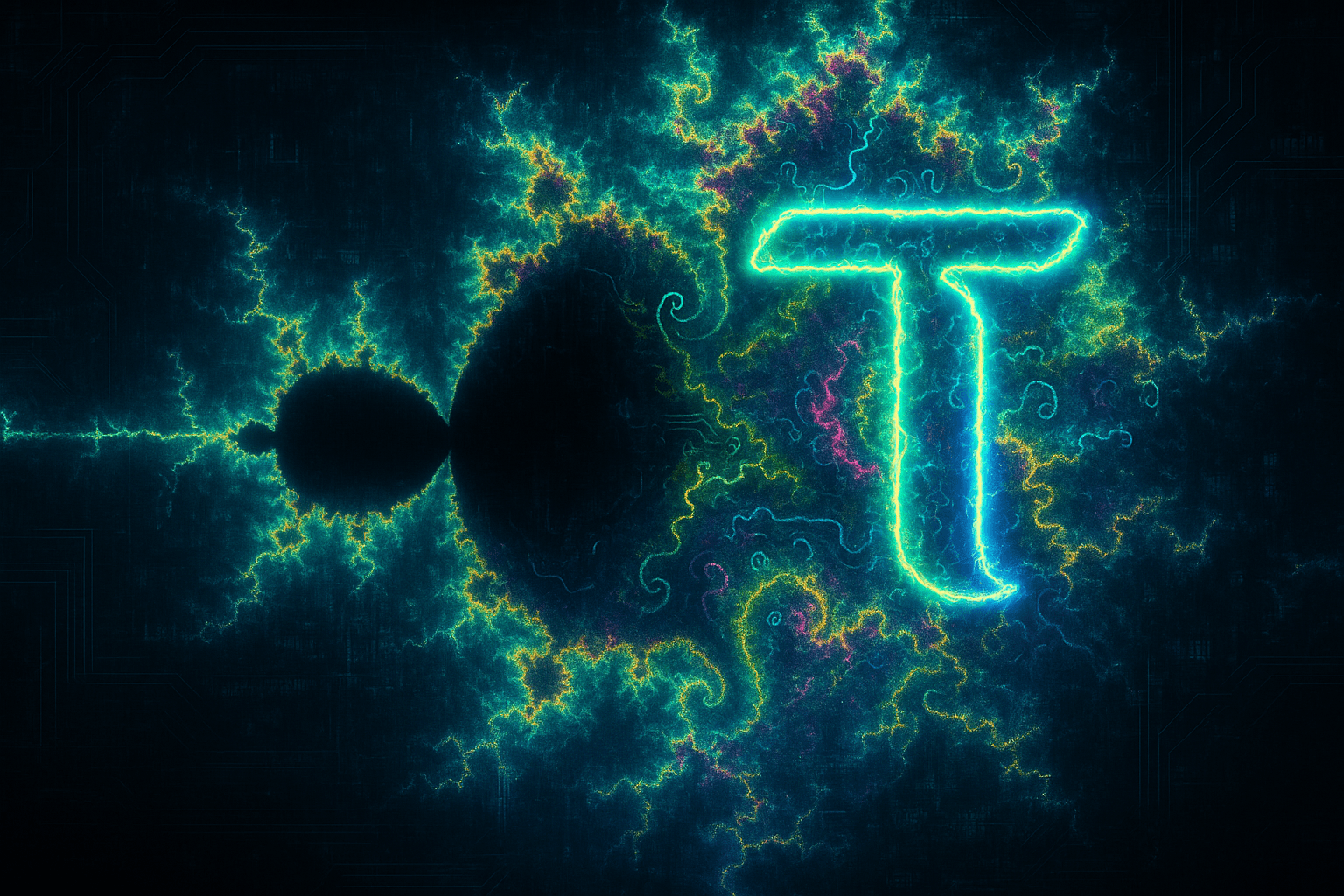

Introduction: Between Order and Chaos
Markets are wild beasts that devour economists for breakfast and quants for lunch. Yet here comes Bittensor, confidently unleashing AI prediction subnets that promise to tame the untamable—a digital oracle network built from machines whispering to each other about tomorrow's prices and probabilities. Networks like SN8 (Proprietary Trading Network) and SN50 (Synth), alongside sports prediction platforms like SN44 (Score) and SN41 (Sportstensor), promise to deliver oracular insights through distributed machine learning. But a fundamental question looms: Are these networks building on solid theoretical ground, or do they rest on mathematical assumptions that may not withstand scrutiny when viewed through the lens of market complexity?
To answer this question, we need a robust framework for understanding markets themselves. And there's perhaps no better intellectual foundation than the revolutionary work of Benoit Mandelbrot—the maverick mathematician who forever changed how we understand financial markets through his application of fractal geometry. His insights provide a powerful lens through which we can critically examine the underlying assumptions and potential limitations of AI prediction models in the Bittensor ecosystem.
Mandelbrot's Vision: Markets as Fractal Systems
Before examining prediction subnets, we must understand Mandelbrot's revolutionary perspective on markets.
Born in Warsaw in 1924 and educated amidst the chaos of wartime Europe, Benoit Mandelbrot developed a uniquely geometric approach to mathematics. His unconventional education—interrupted by war and survival—liberated him from standard mathematical thinking and allowed him to see patterns where others perceived only randomness. During his 35-year career at IBM, Mandelbrot pioneered fractal geometry, a mathematical language capable of describing the irregular, complex shapes abundant in nature but inexpressible through traditional Euclidean geometry.
Three key properties define fractals:
- Self-similarity: Patterns that repeat at different scales, revealing similar structures whether zoomed in or out
- Fractal dimension: Measurements between whole-number dimensions, expressing a complexity that transcends traditional geometric understanding
- Infinite complexity: Endless detail that maintains structured patterns at every level of magnification
When Mandelbrot turned his attention to financial markets, he made a startling discovery: market price movements exhibited fractal properties. In his groundbreaking work "The Misbehavior of Markets," he demonstrated that financial time series display:
- Fat tails: Extreme market events occur far more frequently than normal distribution models predict
- Scaling properties: Similar patterns emerge across different time scales—daily, weekly, or monthly charts exhibit comparable statistical properties
- Long-term dependence: Current price movements may be influenced by distant past movements, contradicting the "memory-less" random walk theory
This fractal view fundamentally challenged the dominant Efficient Market Hypothesis (EMH), which assumes markets reflect all available information and follow a random walk. Instead, Mandelbrot proposed markets exist in a realm between order and chaos—displaying patterns and structure unlike pure randomness, yet remaining fundamentally unpredictable in precise terms unlike deterministic systems.
Bittensor's Prediction Subnets: A Brief Overview
Before applying Mandelbrot's framework, let's examine the key prediction subnets in the Bittensor ecosystem:
-
SN8 - Proprietary Trading Network (Taoshi): This subnet aims to incentivize the creation of profitable trading strategies across various financial markets. Validators assess miners based on their ability to generate returns, rewarding those who consistently outperform market benchmarks.
-
SN50 - Synth: This newer subnet focuses on synthetic data generation for financial forecasting, creating valuable training data that can theoretically improve prediction models across the network.
-
SN44 - Score & SN41 - Sportstensor: These subnets apply similar predictive principles to sports betting markets, attempting to forecast game outcomes and create profitable betting strategies.
All these subnets share a common foundation: they assume sufficient pattern recognition capability, powered by advanced machine learning across distributed networks, can extract predictive signals from noisy market data.
Applying Fractal Market Theory to Prediction Subnets
Mandelbrot's insights offer several critical perspectives for evaluating Bittensor's prediction subnets:
1. The Fundamental Unpredictability of Complex Systems
If markets are truly fractal in nature, they exhibit what mathematicians call "sensitive dependence on initial conditions"—a hallmark of chaotic systems. This means two nearly identical market states can diverge dramatically over time, making precise long-term prediction fundamentally impossible, regardless of computational power or algorithmic sophistication.
Implication for Bittensor: Prediction subnets like SN8 and SN50 may be attempting the mathematically impossible when seeking consistent long-term forecasting accuracy. The fractal nature of markets suggests inherent limitations that no amount of distributed intelligence can overcome.
2. Investment Horizons and Market Stability
The Fractal Markets Hypothesis (FMH), developed by Edgar Peters based on Mandelbrot's work, emphasizes that market stability depends on the diversity of investment horizons. Markets remain stable when participants operate on different timeframes—from day traders to long-term investors. Crises occur when these horizons converge, creating one-sided markets with diminished liquidity.
Implication for Bittensor: Prediction subnets might inadvertently contribute to horizon convergence if they become influential enough. If multiple AI systems converge on similar predictions and timeframes, they could potentially amplify market instability rather than predict it.
3. The Statistical Limitations of Historical Data
Mandelbrot's work on power law distributions in markets reveals that extreme events occur far more frequently than standard models predict. This means historical data may insufficiently capture the true risk profile of markets, particularly during regime changes or crises.
Implication for Bittensor: Prediction models trained primarily on historical data—as most machine learning systems are—may be systematically blind to the most significant market moves. This creates a dangerous paradox: the very events most valuable to predict are those the systems are least equipped to anticipate.
4. Volatility Clustering and Local Predictability
While Mandelbrot challenged the notion of efficient markets, he did identify phenomena like volatility clustering—periods of high volatility tend to follow other high-volatility periods, and calm periods follow calm. This suggests a degree of local predictability within the broader unpredictable structure.
Implication for Bittensor: Prediction subnets might find more success by focusing on volatility forecasting and risk management rather than price direction prediction. By acknowledging the fractal nature of markets, these networks could reorient toward more achievable goals.
The Future of Prediction Subnets: Between Hubris and Innovation
Mandelbrot's work doesn't suggest we should abandon market prediction entirely. Rather, it invites a more nuanced approach that embraces complexity instead of hiding from it. For Bittensor's prediction subnets to mature beyond selling "smoke and mirrors," they might consider several shifts in focus:
1. From Point Predictions to Probability Distributions
Rather than attempting precise price targets, prediction subnets could generate and refine probability distributions of possible outcomes. This approach acknowledges the fundamental uncertainty in complex systems while still providing valuable information.
2. From Universal Models to Adaptive Frameworks
The fractal nature of markets suggests they may undergo phase transitions—periods where the underlying statistical properties themselves change. Successful prediction subnets might focus on detecting these regime changes rather than assuming constant market behavior.
3. From Performance Metrics to Risk Management
Instead of rewarding validators solely based on prediction accuracy or trading returns, networks could incorporate sophisticated risk management criteria. This would align incentives with the reality of market uncertainty.
4. From Competition to Diverse Perspectives
The strength of distributed networks lies not in finding a single "best" prediction but in maintaining diverse analytical perspectives. By explicitly rewarding methodological diversity, prediction subnets could build resilience against the convergence that leads to market instability.
Conclusion: The Wisdom of Uncertainty
Are Bittensor's prediction subnets selling smoke and mirrors? Through Mandelbrot's fractal lens, the answer is nuanced. To the extent they promise consistent, precise market predictions, they likely oversell their capabilities—no system, however sophisticated, can overcome the fundamental unpredictability of fractal systems.
However, to the extent they embrace and quantify uncertainty, detect regime changes, manage risk, and maintain analytical diversity, they may offer genuine value. As Mandelbrot himself observed, "A fractal is a way of seeing infinity." Perhaps prediction subnets are best viewed not as oracles but as tools for navigating complexity—acknowledging the infinite detail of markets while recognizing the patterns that emerge across different scales.
The ultimate test for SN8, SN50, and other prediction subnets will be whether they can incorporate the humility before complexity that was Mandelbrot's most valuable legacy. In a world increasingly dominated by algorithmic trading and AI-powered forecasting, this recognition of the limits of prediction may be their most important contribution to the future of decentralized intelligence.